Table of contents
Google Ads campaigns have many elements that affect their effectiveness. However, drastic changes in campaigns can cause concerns of the „what happens when” kind and the inability to compare the behavior of campaigns in real time under different settings, even more so considering changes in the industry such as seasonality, competitiveness and currently ongoing events. What if I said there was a solution for this?
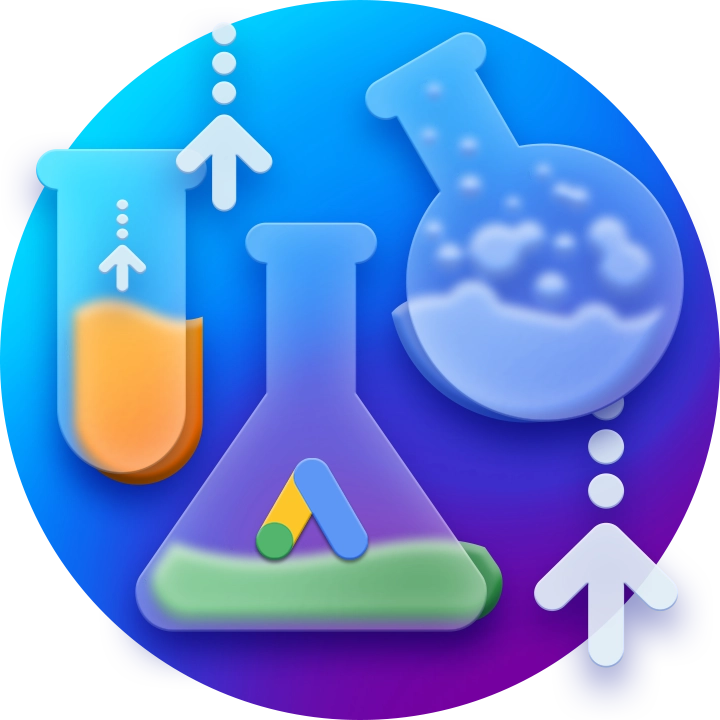
Google Ads Experiments allows you to conveniently test the new settings in an automated test, splitting traffic from the campaign into the original campaign and the experimental campaign according to the proportions we set – preferably in a 50% split. After obtaining enough data to make the results reliable, such a test can be terminated or the tested changes can be implemented – depending on the results achieved for each variant. This is a particularly valuable functionality when we are already sure that the structure of the advertising account itself can no longer be improved in a significant way, we are satisfied with the phrases and exclusions we have chosen, and we want to test changes on a macro scale. Especially since the relevance of the change is a very important factor in the design of the experiment.
Conducting such an experiment has so far gone through a two-step process – first we had to create a draft version of the campaign to make all the changes to be compared, and only after it was set up did we launch the experiment. This is no longer necessary – Google has simplified the process and provided additional options for experiments, for all of which you can already determine which campaigns they apply to when creating an experiment and create a trial campaign through this interface. Naming an experimental campaign has also been made easier – just add your own suffix, and it will be added automatically to the name derived from the original campaign.
What is worth testing?
There are quite a few possibilities, not the least of which is to exercise moderation in the selection of variables – because the more elements that differentiate the main campaign and the experiment, the more difficult it is to attribute changes in statistics to a specific factor.
Experiments, for example, can be used to test changes in keywords, landing pages, rate-setting strategies, audience groups and other elements. By testing a change on only a portion of the traffic, you risk less and in a controlled way.
How, on the other hand, does this look like in practice? Through experimentation you can find out:
- whether a change in an ad’s headline affects the results achieved-and other content changes of a similar nature.
- whether changing the landing page translates into more conversions – it may turn out that where on the site a potential customer lands will significantly determine whether he or she will perform the desired action on the site.
- how changes in display URLs translate into results – worth noting especially since this is one of the few fields in flexible search ads that doesn’t allow you to add multiple variations to the rotation.
- how changing the keyword pool and matching affects campaign performance – this might be a good opportunity to test approximate keyword matching if we have concerns here about expanding reach and allowing algorithms that much freedom.
- whether extending targeting to new geographic areas will yield a positive result – this will allow us to assess the potential of campaigns in new regions, while retaining those that are already working well.
And these are just a few examples of experimentation scenarios. However, it is important to keep in mind that we are deprived of some functionality in the experiments. We won’t see search phrases, auction analysis – so before we do such a test, let’s make sure we’ve definitely covered all relevant exclusions.
Nevertheless, due to the limitations of experiments, when choosing a tested element, it is worth thinking about its real importance – whether a given change is likely to bring tangible results at all?
And there are 3 types of experiments available:
- relating to changes in text ads-
- experiment based on different video ads (in this case, within one experiment, we can define from 2 to 4 variants)
- a custom experiment, similar to the previous ones, where it is already up to us which exact setting we will change – although the last option is only available for search and ad network campaigns.
The very nature of the search engine campaign additionally allows us to decide whether to divide the traffic in the experiment based on searches or cookies – in the former case, each search is newly assigned the main or test version of the campaign – in the latter, the assigned version is assigned to a person and no longer changes regardless of the number of queries made. However, this requires a sufficiently large list of users, so in this case we are talking about campaigns with a rather larger volume of traffic.
What is worth remembering?
Campaign allocation is based on the number of auctions and not the budget, so it may happen that different versions spend budgets in different amounts, especially if their effectiveness varies significantly. This is not a cause for concern, but the natural course of things.
The experiments do not use historical data of the original campaigns, so the first two weeks of operation should not be taken into account when comparing results – this is a standard learning period for algorithms. I therefore recommend allocating at least 6 weeks for such a test. In addition, each experiment can start at the earliest the next day – to always have full results from the whole day. It is even good practice to set it up well in advance, so that any delay in the acceptance of a new campaign does not disrupt the test.
Complex test programs also require effective scheduling. You can set as many as five of them in advance against a single campaign, but you can only have one active experiment at a time, so the key is to choose the variables of greatest importance.
If you want to test changes in a campaign that shares a budget with others, you will have to separate them first – it is not possible to run experiments on campaigns with a common budget.
It is quite important to set the end date of the experiment to an even further date than we initially anticipate – mainly because while an ongoing experiment can be interrupted at any time, an experiment that has already ended cannot be extended. An already running experiment cannot be temporarily paused either, it will continue to run until it is interrupted or terminated.
We should also be wary of modifications to the original campaign during the test – while it is true that Google already allows for synchronization between the main campaign and the experimental campaign when creating the experiment, if such changes take place, nevertheless this can disrupt the results, just as it would change the performance of the campaign and without the experiment.
Another important point is that experiments are done at the campaign level – if we want to test a more global change – such as the effect of a landing page on results – at the level of the entire account, unfortunately this will require setting up an experiment for each campaign separately. It is then wiser to start with a select few campaigns and, based on their testing, make a decision for the entire structure of the advertising account.
We already have the data, and what’s next?
Depending on the results (and, based on them, our decision on what to do next), you can end the experiment without making changes, apply the changes to the existing original campaign, or create a new campaign from the experiment. The last option seems most appropriate if the changes made want to cut off with a thick line from what has gone before.
Google statistics present in a clear way to what extent the changes in the selected parameters are statistically significant. However, it may happen that despite the longer duration of the test, the experiment did not get enough reliability. This could be due to the low intensity of the campaign – and thus too little data – or the fact that the change made does not affect the campaign as significantly as we would expect.
In the new experiment interface, we already have the ability to tailor the results report to our expectations – and include those statistics that are most relevant to us, and that we want – through the experiment – to improve.
When analyzing the data, it is also worth checking whether the results obtained apply evenly to the entire campaign, or whether, depending on a particular group of ads, they differ significantly – and take this into account when making modifications.
One experiment once in a while will also not bring as much results as consistent, regular testing of new ideas for improving the campaign – each percentage improvement in the result can eventually accumulate to impressive proportions.
In conclusion, experimentation in Google Ads is definitely a game worth the candle. A good practice digital agencies that will benefit everyone involved – is to document the results from experiments already conducted. This will allow, for example, that when creating new campaigns within the same account, you can immediately take into account the conclusions already developed.
It also looks like Google will be more and more willing to offer solutions in this area to embolden hesitant advertisers to back up their algorithms’ superiority with real data, relating specifically to a given business. Already, test solutions covering more campaign segments are emerging. Perhaps in time, Discovery and PLA campaigns will also see tests with a similar multiplicity of options.
What’s more, it’s worth taking an interest in what additional tools offer on this topic to make working on campaigns easier, especially if you already use them for other purposes. For example, Optmyzr allows you to see multiple experiments on different ad accounts at once – making it easier to control them and draw conclusions. Have a successful and fruitful testing experience!
Was the article helpful?
Rate our article, it means a lot to us!
Let's talk!
Certified specialist with many years of experience, with Up&More since 2016. Her campaigns have been awarded many times . She has experience with clients from the development, automotive and mobile application industries.